AI in Cybersecurity has been among the most valuable features of the digital age. Cyberattacks have grown more advanced and frequent by the year. Consider, for example, the WannaCry ransomware attack in 2017, which paralyzed systems in over 150 countries—from healthcare to finance. Such an incident indicated the urgent requirement for more advanced, scalable, and intelligent solutions to tackle emerging cyber threats.
Those were robust systems some time ago; however, as of today, they’re just not enough to enhance threats. Hackers depend on more strategic methodologies, such as polymorphic viruses, phishing emails, and attacks using artificial intelligence. Consequently, many companies have recently considered AI a game-changer, which will increase their position in the security strength scale.
AI has revolutionized cybersecurity. It allows for real-time threat detection, forecasting potential future vulnerabilities, and automatic responses so that damage can be reduced to minimal amounts. The blog looks at how AI is revolutionizing the landscape of cybersecurity, from current trends and real-world applications to challenges with integrating AI in cybersecurity systems.
The Cybersecurity Landscape Today
Changing Nature of Threats
Cyber threats today are more complex and vicious than ever:
Ransomware: Ransomware attacks have now become a multi-billion-dollar criminal business. The 2021 Colonial Pipeline attack stopped fuel supplies in the U.S., forcing the company to pay millions in ransom.
Phishing and Social Engineering: Cybercrimes use phishing emails and impersonation tactics to hoodwink people into revealing confidential information. Recently, in 2020, audio was used to impersonate the CEO of a company to deceive the company into transferring $243,000 to fraudsters.
Polymorphic Malware: is malware that constantly changes its code. Thus, it evades detection and can’t be easily caught by traditional signature-based systems.
Limitations of Conventional Security Control
Traditional security control is well at work in the fight against known threats but has become inefficient against the complexity and volume of modern threats:
Static Defense Mechanisms: A signature-based system leaves out new or changing threats, such as zero-day vulnerability.
Processes Involving Manual Monitoring: Humans struggle to keep track of the vast number of alerts, causing alert fatigue and missed detection.
Scalability Challenge: Digital infrastructures are growing, thus more advanced systems are needed to secure large-scale networks.
These constraints highlight the importance of AI-based solutions, which can learn, adapt, and respond to threats in real-time.
How AI is Revolutionizing Cybersecurity: Threat Detection and Prediction
AI can detect threats by analyzing large volumes of data and establishing patterns and anomalies.ML models can predict attacks based on past behavior.
Anomaly Detection: AI-based systems create a baseline of normal behavior and raise alerts in real-time when something deviates from the norm.
Predictive Analytics: Using AI, strength and attack trends of vulnerabilities are analyzed in advance to take proactive measures, such as patching vulnerabilities before exploitation.
Malware Detection and Zero-Day Threats
Malware detection is enhanced through behavior rather than code signatures, making it highly effective against polymorphic malware and zero-day threats.
Behavioral Analysis: It evaluates files’ behavior in isolated environments and identifies malicious intent without pre-existing signatures.
Real-Time Response: AI-driven systems can instantly detect and neutralize threats, preventing damage from spreading across networks.
Securing Networks
AI strengthens network security through automated monitoring, intrusion detection, and response systems.
AI-Driven Intrusion Prevention Systems (IPS): These systems analyze network traffic, identifying and mitigating threats before they cause harm.
Autonomous Isolation: Compromised devices can be automatically quarantined to prevent further breaches.
Social Engineering Mitigation
Social engineering attacks like phishing are among the most difficult to detect. AI uses advanced tools to fight these threats:
Natural Language Processing (NLP): AI in data security analyzes email content to detect phishing attempts, flagging suspicious language or sender behavior.
Deepfake Detection: AI algorithms identify inconsistencies in video and audio files, mitigating the risk of impersonation.
Real-World Applications of AI in Cybersecurity
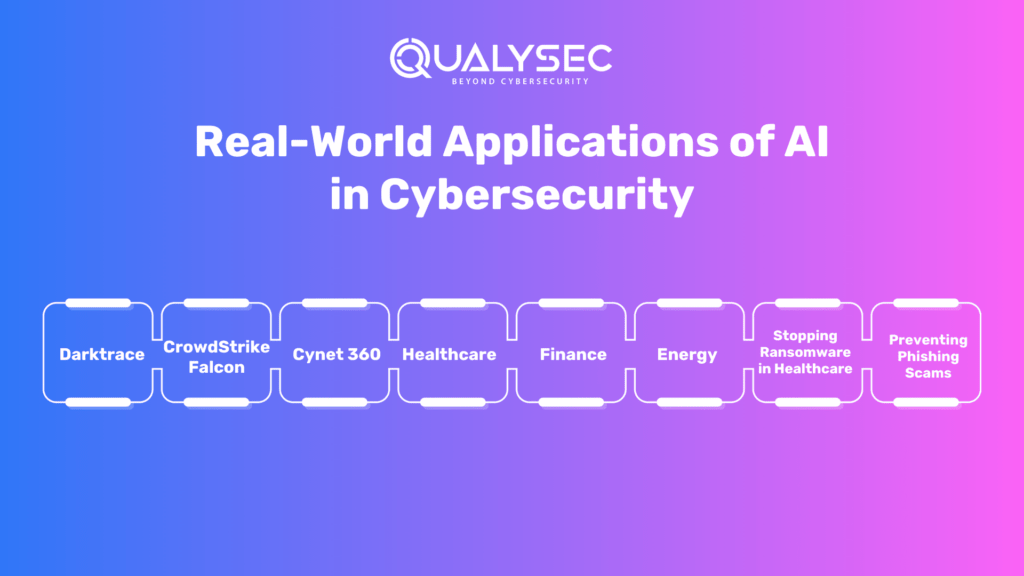
AI-Powered Tools in Use
Several tools illustrate the strength of AI cybersecurity:
Darktrace: Leverages unsupervised learning to understand network behavior and detect deviations.
CrowdStrike Falcon: Provides the amalgamation of endpoint protection along with real-time threat intelligence to further enhance security.
Cynet 360: Offers an integrated platform to protect endpoint, user, and network systems.
Industry-Specific Use Cases
Healthcare: AI and cybersecurity protect patient records against ransomware attacks while complying with data protection regulations.
Finance: Fraud detection systems use AI to monitor any transactions containing defects and bring them to the system before escalation occurs.
Energy: AI protects critical infrastructures like power grids and oil pipes against cyber attacks.
Case Studies
Stopping Ransomware in Healthcare: A US-based hospital’s use of AI-based tools detected unusual encryption activity, and thus, the ransomware attack was checked at its earliest stage.
Preventing Phishing Scams: An AI-based email security system helped a financial firm reduce phishing-related incidents by 85%.
Emerging Trends in AI-Powered Cybersecurity
Federated Learning for Privacy-Sensitive Threat Detection
Federated learning allows organizations to collectively train AI models without sharing sensitive data. This decentralized approach enhances AI information security without violating data privacy.
Quantum-Resistant Security
Quantum computing has become a significant threat to encryption methods. AI significantly contributes to developing quantum-resistant algorithms and securing data in the post-quantum world.
Biometric Authentication
AI improves authentication systems by integrating multiple biometrics, including facial recognition, fingerprint scanning, and behavioural analysis.
Example: Banks and financial institutions are using voice recognition and AI-driven behavioral analytics to verify and authenticate customers with security and fluidity.
AI-Driven Automation in Incident Response
The SOAR solution uses AI to prioritize and automate responses to threats and reduces downtime as well as the associated operational costs significantly.
AI with Blockchain
The combination of AI with blockchain is advantageous because it provides increased transparency and security. The blockchain logs are tamper-proof, and AI can scan the data for any abnormalities. In this manner, an effective defense mechanism is obtained.
Latest Penetration Testing Report
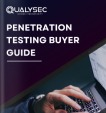
Challenges and Limitations of AI in Cybersecurity
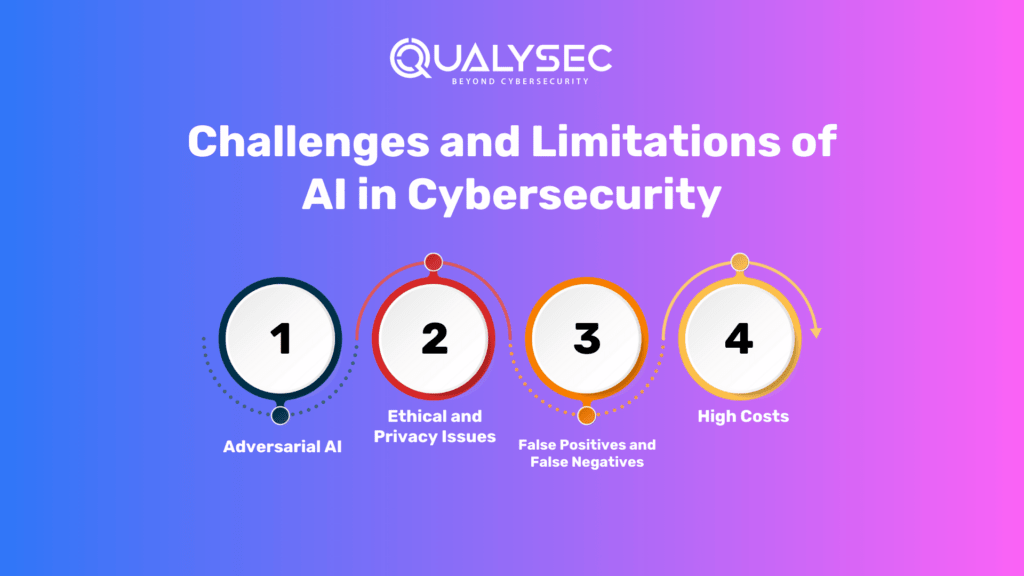
AI has revolutionized the cybersecurity landscape. However, this technology has challenges and limitations. Understanding these concerns is essential to balancing the use of AI with its potential drawbacks.
1. Adversarial AI
Cybercriminals have discovered that using AI is a huge threat. These attackers use adversarial AI techniques to bypass sophisticated security systems. For example, phishing emails generated using AI can be extremely hyper-personalized by scrutinizing the target’s online activities, preferences, and behavioural patterns. These messages are nearly undetectable because they appear exactly like legitimate communications in traditional systems. Moreover, hackers also use AI to design malware, which can learn and evolve, avoiding mechanisms that depend on static signatures. This dynamic nature requires continuous innovation to effectively combat adversarial AI cybersecurity threat tactics.
2. Ethical and Privacy Issues
AI data security can feed on large volumes of data, thus raising ethical questions about how such data is collected, stored, and used. Often, organizations must process sensitive personal information in training AI models effectively. However, the dependence on data would place a risk of misuse, breaches, and privacy violations.
Furthermore, it is challenging to strike a balance between innovation and ethics. For example, organizations may need to anonymize or encrypt data to ensure privacy, but this may not be helpful for AI in terms of pattern analysis. Ethical issues also involve transparency and accountability of AI-driven decisions, where stakeholders want clearer insights into how such systems work.
3. False Positives and False Negatives
artificial intelligence in security systems are not perfect, and they are prone to mistakes like false positives, which means something benign gets misidentified as a threat, and false negatives, which fail to make a real alert when the action is going on.
False Positives: It flags innocuous activities as malicious, resulting in unnecessary alerts that bombard the security teams. Such a phenomenon as alert fatigue causes critical alerts to be missed, reducing overall effectiveness.
False Negatives: On the flip side, if an AI system fails to detect a legitimate threat, the organization remains vulnerable to attacks that go unnoticed until it’s too late. Such gaps in detection can have disastrous consequences, especially in industries handling critical infrastructure or sensitive data.
Improving the accuracy and reliability of AI algorithms is vital to minimize these risks.
4. High Costs
It also requires several new investments to set up AI-powered cybersecurity solutions. An organization must invest in acquiring the latest infrastructure, training AI models, and maintaining these systems.
Infrastructural Costs: Implementing the foundational hardware and software for an AI system is usually expensive. Cloud-based solutions might reduce costs, but they certainly involve recurrent expenses.
Training and Experience: Properly designing and implementing efficient AI models requires experience, which may be a bottleneck for smaller firms with limited financial resources.
Ongoing Support: Updating, retraining models, and dealing with unexpected problems also increase the overall costs of operating AI systems over time.
The above financial limitations make AI-based cybersecurity solutions infeasible for SMEs; instead, they rely on conventional, not-so-efficient techniques.
Addressing such challenges will improve the integration of Cybersecurity in AI strategies into an organization’s most powerful and equitable defence mechanisms.
Human and AI Collaboration in Cybersecurity
Why AI Needs Human Expertise
While AI is excellent at processing data and identifying patterns, human expertise is necessary for:
Training Models: Quality data is the prerequisite for good AI performance.
Strategic Oversight: Humans interpret AI-derived insights to generate effective security solutions.
Ethical Decision-Making: Experts check that ai driven cyber security observes privacy and other ethical considerations
Example of Collaboration
Incident Response Teams: Human analysts investigate suspected anomalies detected by AI.
Human-AI loop for continuous improvement: Feedback loops to refine algorithms.
To further flesh out the piece or make it more detailed, you can incorporate these sections below:
AI in Cyber Threat Intelligence
Let’s explore how AI improves cyber threat intelligence by filtering global threat data and giving actionable insights.
Threat Hunting: The art of how AI cybersecurity systems identify potential adversaries with their tactics.
Global Intelligence Sharing: AI-enabled systems, like IBM X-Force Exchange, where organizations can share threat data securely.
Proactive Security Measures: How AI stops attacks through shared intelligence.
Ethical AI in Cybersecurity
Go deeper into ethical considerations that have to do with AI in cybersecurity:
Bias in AI Models: How biased data might malfunction in threat detection.
Transparency: Explainability in AI Models of critical systems
Regulation: The new legislations and regulations that come into play impact the ethical use of Artificial intelligence in cyber security.
Cybersecurity using AI for SME’s
This section discusses how SMES, with usually very thin resources, can adapt to the budget-friendly AI tool.
Budget-Friendly AI Tools: Give examples such as Cylance Smart Antivirus, or other cheap tools.
Challenges for SMEs: Discuss challenges of implementation and scalability.
Success Stories: Present success stories of SMEs using AI to fight against cyber threats.
Future of AI in Cybersecurity
Present possible innovative improvements and what AI holds for the long term in the realm of cybersecurity:
AI in Autonomous Systems: The potential of complete autonomous cybersecurity operations.
AI and IoT Security: Securing Smart devices through advanced AI models.
AI and Cognitive Computing: Understanding how AI could simulate human thinking to make better security decisions.
AI-Powered Cybersecurity Training
Describe how AI is revolutionizing cybersecurity training and education:
Simulated Environments: AI-based simulations for employee training in phishing or social engineering detection.
Skill Gap Reduction: Leveraging AI to build customized learning plans for cybersecurity professionals.
Comparison of AI Cybersecurity Solutions
Compare the different AI-based cybersecurity tools.
Features and Usability: Key features of leading platforms, Darktrace, CrowdStrike, or Palo Alto Cortex.
Scalability: How these tools respond to the needs of enterprise-level companies vs. small businesses.
Customer Success Metrics: ROI or effectiveness when measured in real-world usage.
Role of Government and Policies in AI Cybersecurity
How the government is using AI to keep infrastructure safe and able to combat cyber-crimes.
National AI Cybersecurity Strategies: U.S., China, and EU.
Public-Private Collaboration: How governments and tech work together to further AI in Cybersecurity Market.
Talk to our Cybersecurity Expert to discuss your specific needs and how we can help your business.
Conclusion
However, to unlock AI’s full potential, challenges like adversarial AI, ethical dilemmas, and high implementation costs must be overcome. Human experts will continue to be crucial in conjunction with AI systems to build a secure digital future.
The more AI technology develops, the more it will become part of cybersecurity, offering hope for a world in which digital innovation thrives without compromising security.
0 Comments